Panel Discussion at Hartford AI Day – April 23, 2025
Panelists:
- Moderator: Paul Tyler, Head of Marketing Innovation at Zinnia
- Panelist: Dan Gremmell, Chief Data Officer, Zinnia
- Panelist: Manu Mazumdar, Head of Data Analytics & Insurance Technology, Conning
- Panelist: Imad Eid, SVP Head of Salesforce Technology, Global Atlantic
- Panelist: Doug Elfers, VP, Data Science, Prudential
Introduction
Paul Tyler: I’m Paul Tyler. I work for Zinnia now. Today’s topic focuses on the “so what” panel. How is AI really going to change the industry that we’re all so proud to be in here in Hartford?
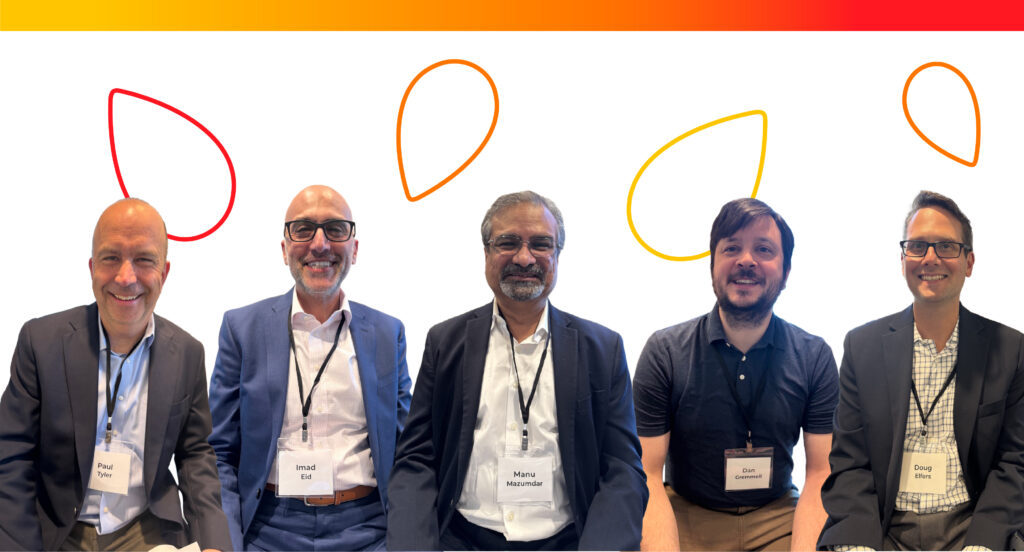
Recently, the CEO of Shopify put out a manifesto with three key points that caught a lot of attention:
- Everyone in the company is expected to use AI for productivity
- Find the people in your organization who are using AI to be 100x more productive
- Before filling a new position, you must justify why AI couldn’t do the job
In our business, where we’re pushing, moving, and transforming data, you’d think our industry would be ripe for AI transformation.
Panelist Introductions
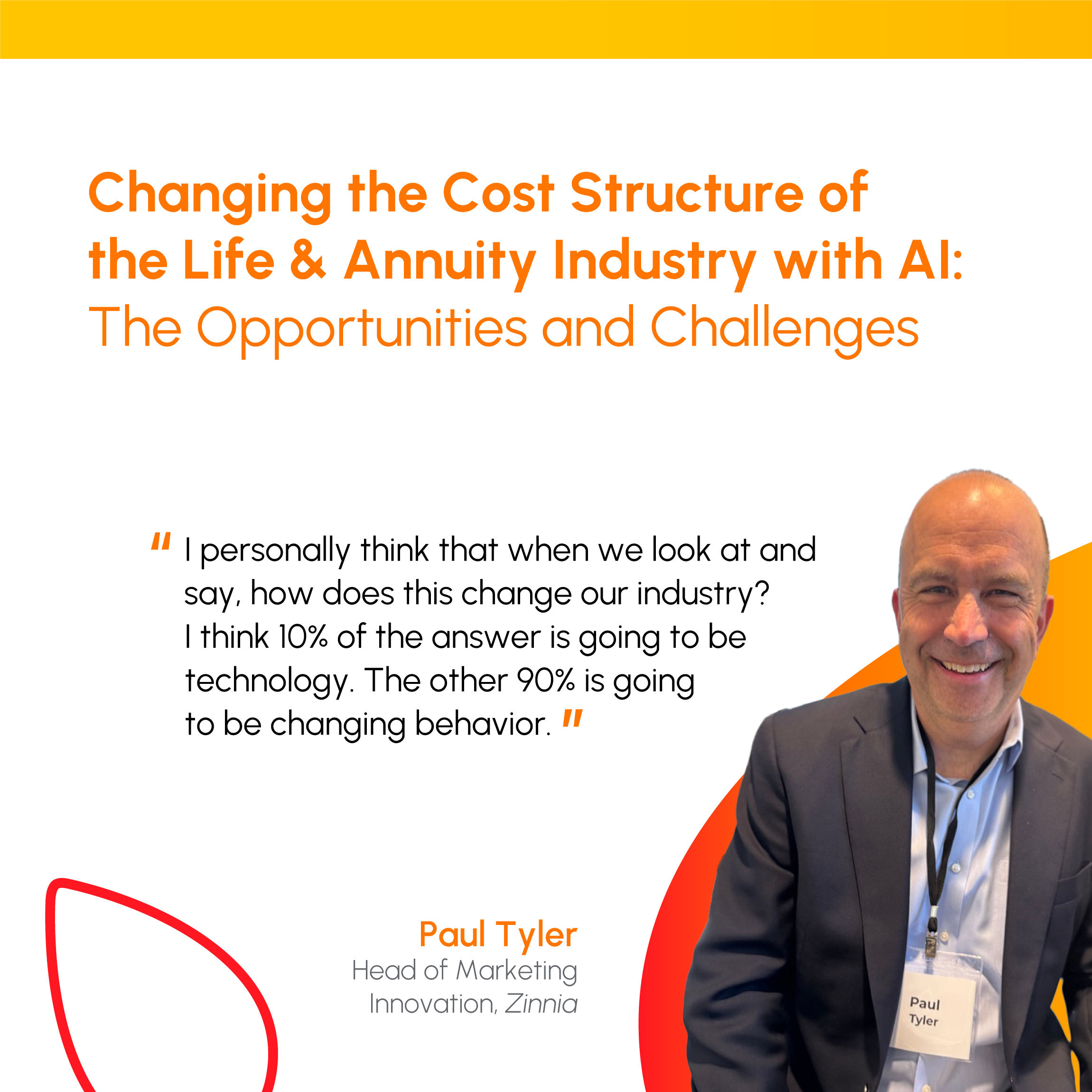
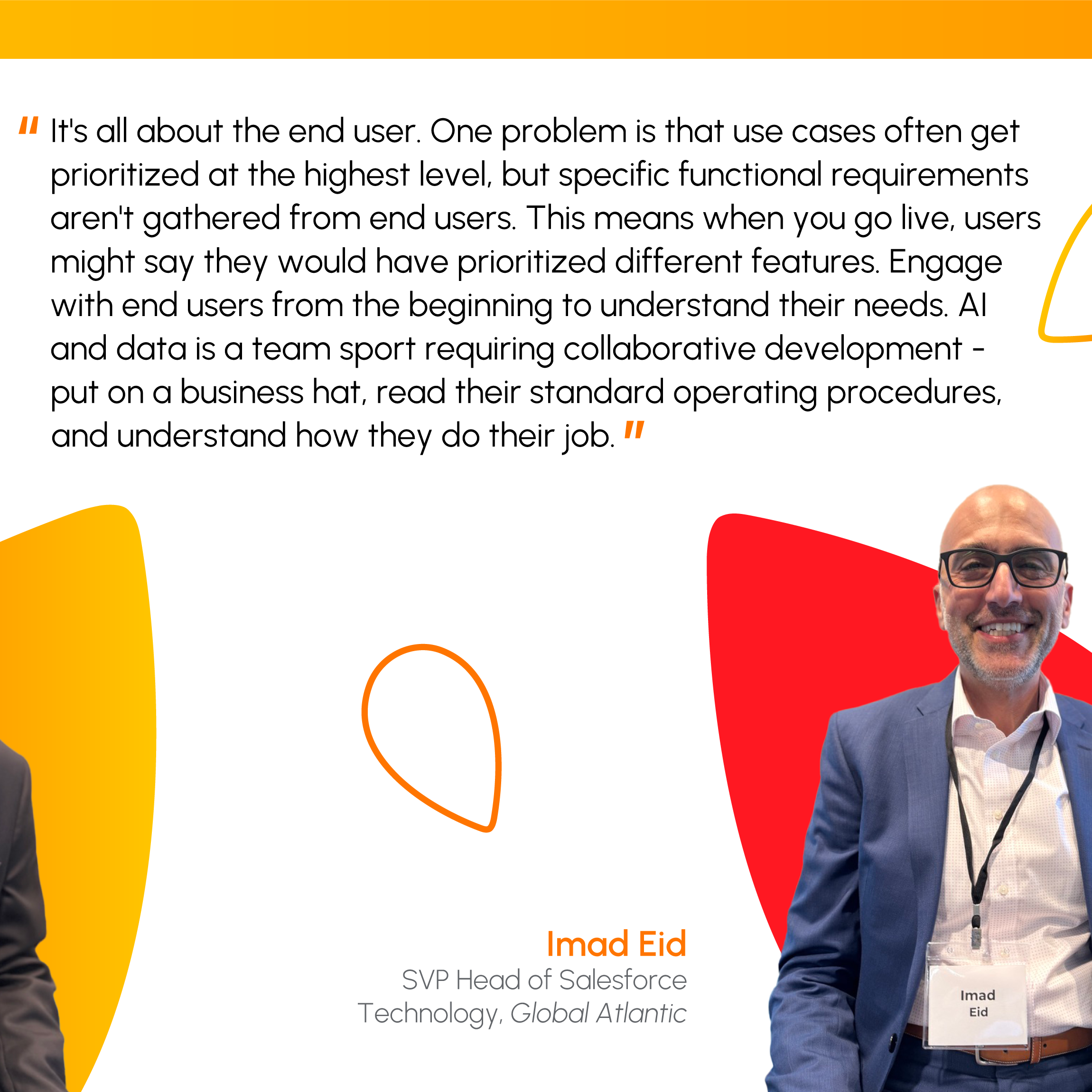
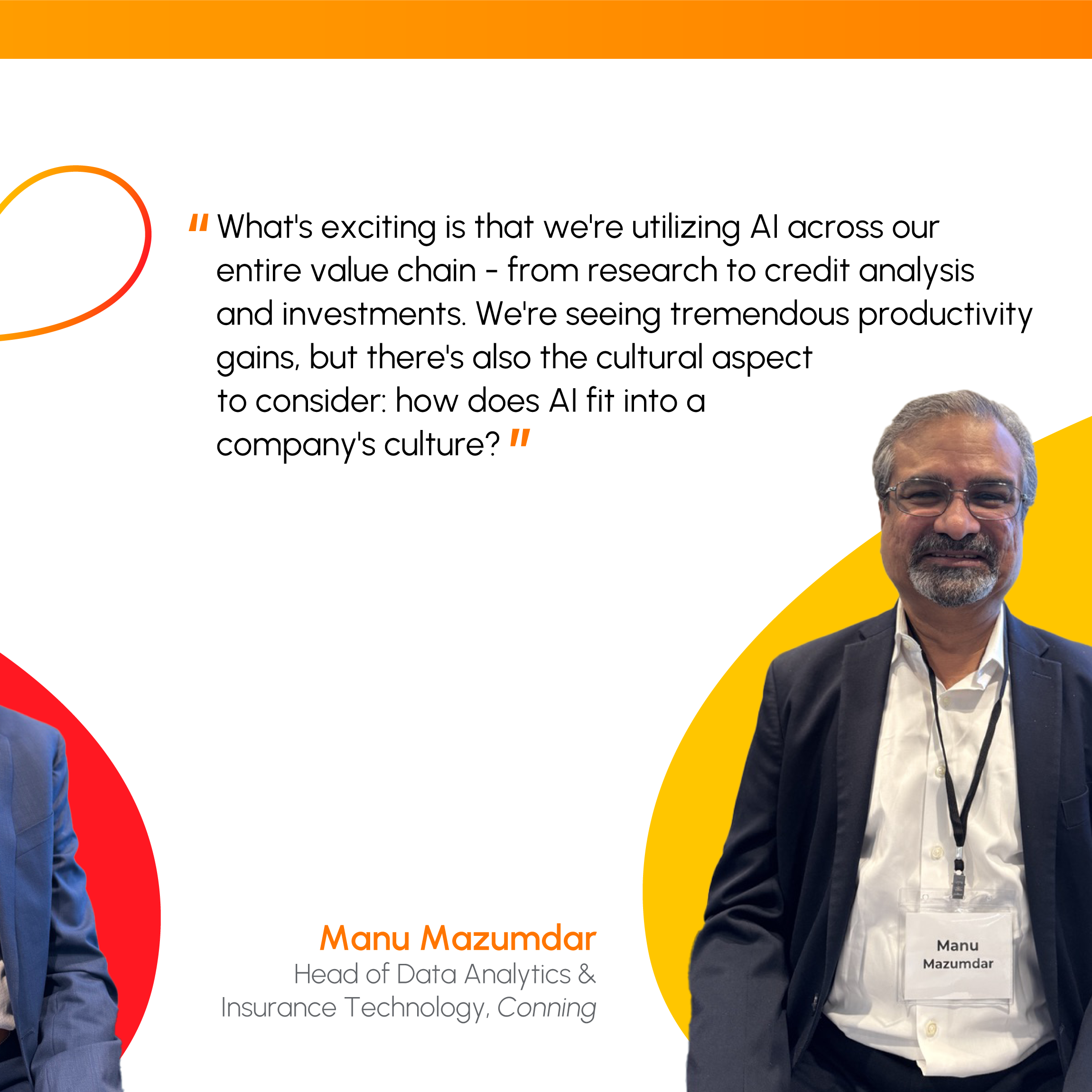
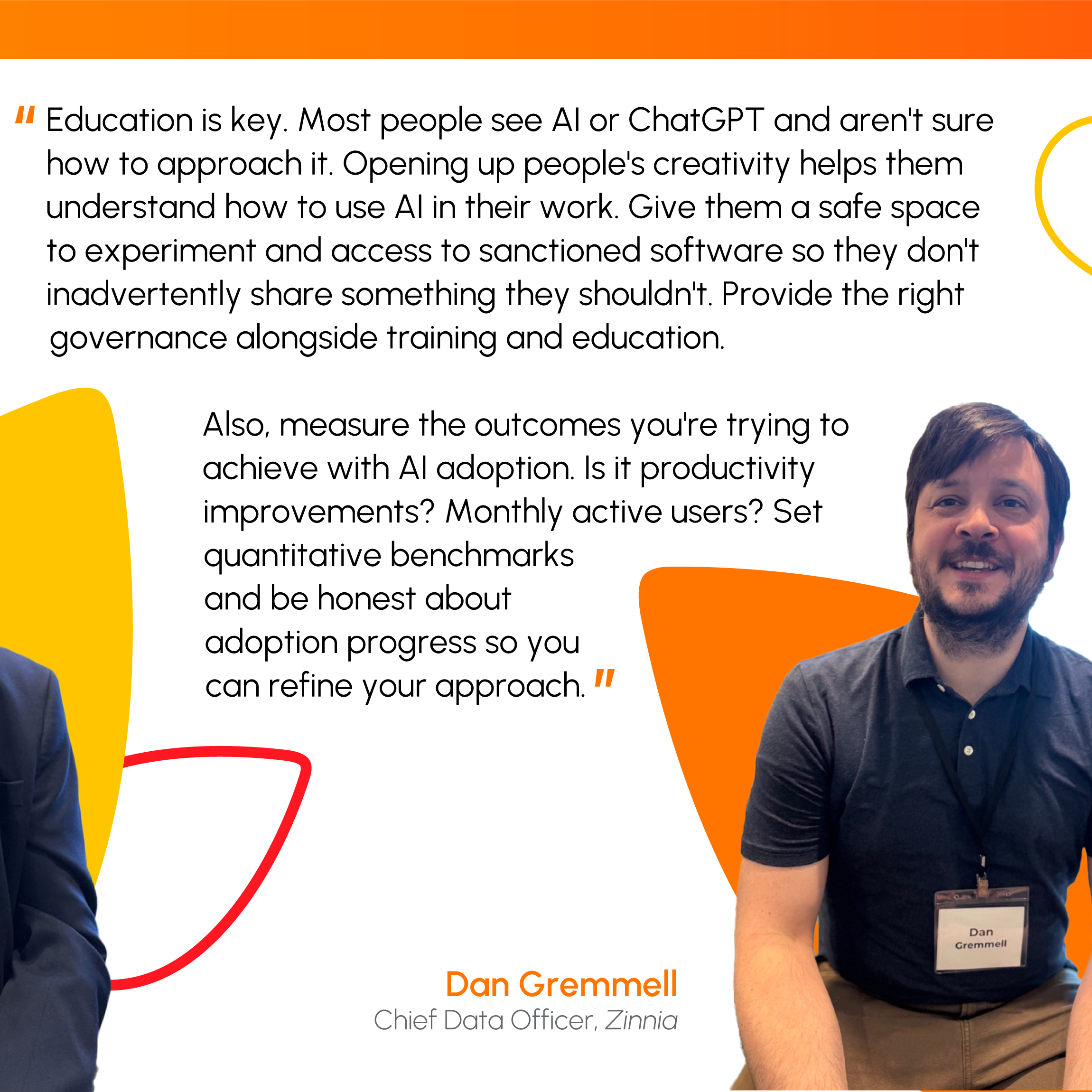
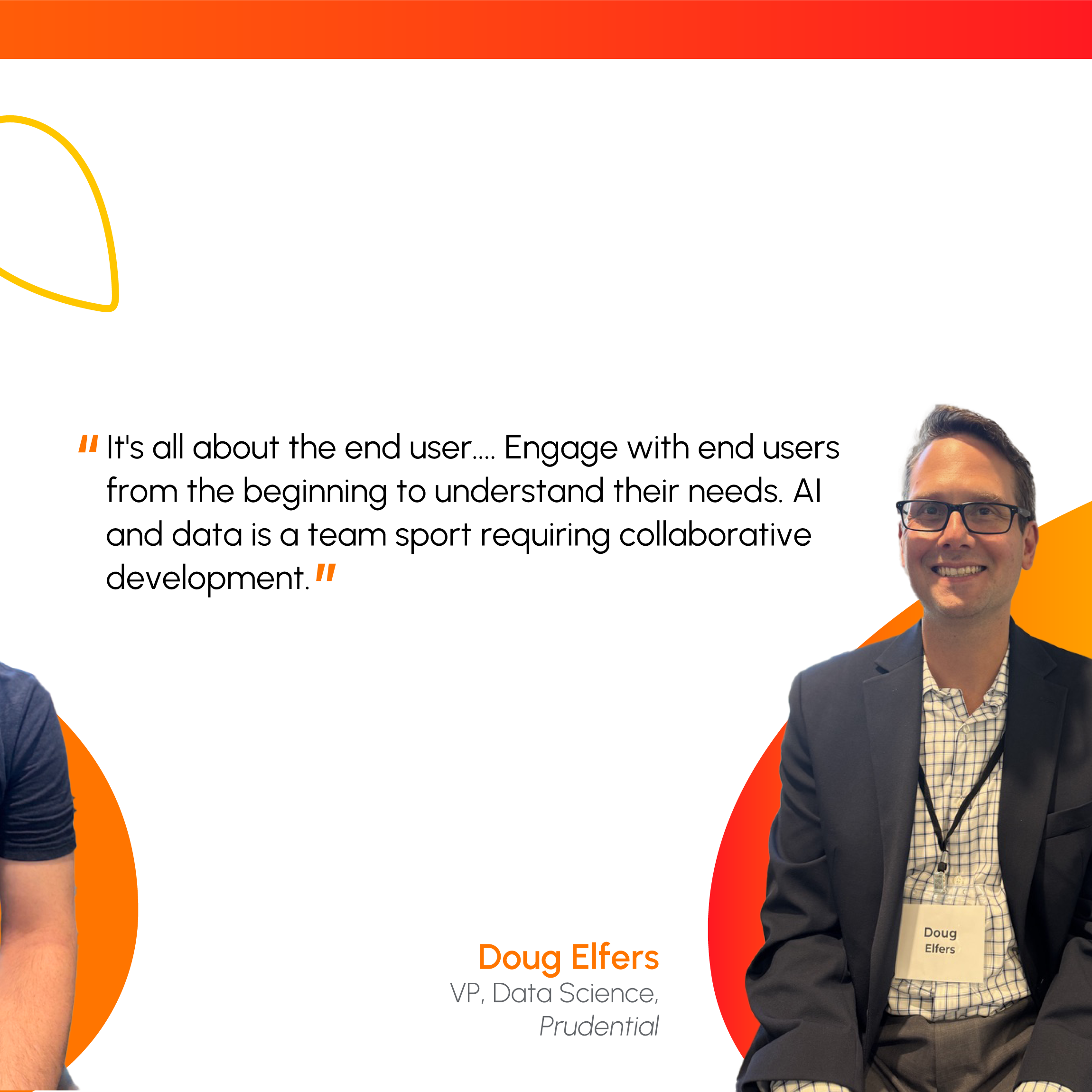
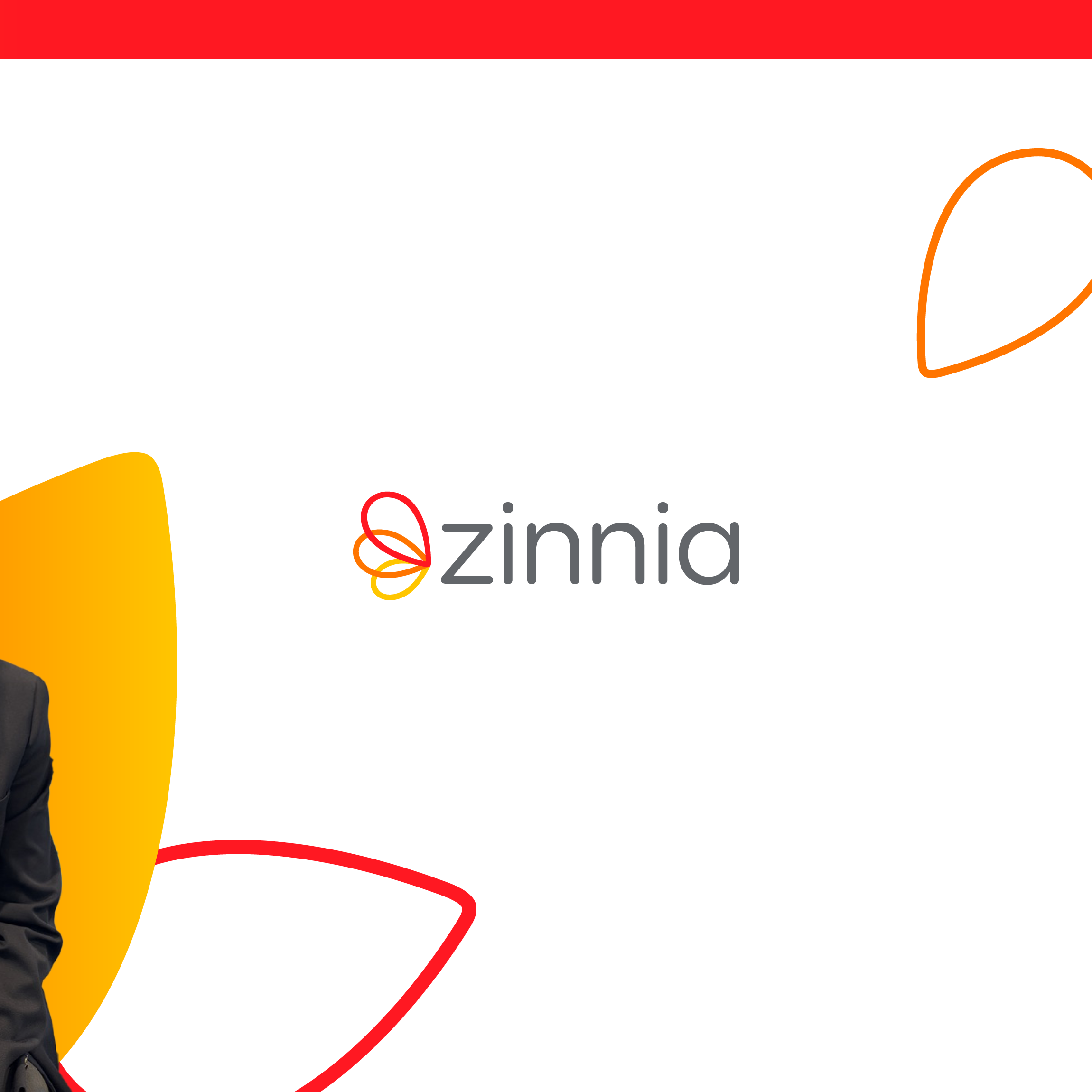
Imad Eid: Hi everyone. I work for Global Atlantic, part of KKR. I’ve been with the firm for over four and a half years and lead the Salesforce practice. Our AI journey started a few years ago when ChatGPT became popular. We’ve implemented predictive AI use cases that are now in production. We’re exploring different AI use cases with various partners in the InsurTech space and beyond. I’m focused on helping our sales organization meet ambitious goals to double our AUM without significantly increasing headcount. AI will be a key player in achieving this.
Manu Mazumdar: I’m the head of data analytics and technology at Conning, an affiliate of Generali Investment Holdings. Conning manages about $200 billion of insurance company assets. I’m also on Conning’s AI Governance Committee. What’s exciting is that we’re evaluating AI across our entire value chain. We’re seeing tremendous productivity gains, but there’s also the cultural aspect to consider: how does AI fit into a company’s culture?
Dan Gremmell: I’m the chief data officer at Zinnia. I lead our data, AI, and machine learning operations. Since GenAI emerged, we’ve done a lot to push it through our contact center, using it to interpret calls and provide quality scores. Before GenAI, we did extensive machine learning with propensity models and prospective value models to optimize our value chain. We still develop these specific machine learning cases regularly, even though people focus more on GenAI now. What excites me most is how GenAI has helped normalize the knowledge spectrum. I can now be a full-stack coder when I might have struggled with that before or needed extensive research. It’s an amazing breakthrough that helps extend skill sets beyond what you already know.
Doug Elfers: I lead the retirement strategies data science and machine learning team at Prudential. I previously led the group insurance and long-term care team. I’ll focus on three domains where we’re applying AI in the individual annuity area:
- Pre-sales enablement: We use traditional machine learning for advisor shortlisting and segmentation. With GenAI, we’re focusing on helping wholesalers spend more time selling by automating administrative tasks. Use cases include:
- Automated administrative emails
- Quick record updates
- Call summarization with real-time intelligence
- Meeting preparation that pulls relevant data from multiple sources
- Post-meeting activity capture that automatically transcribes notes and sets up next actions
- Purchase experience: We’re focusing on straight-through processing using intelligent document processing and GenAI tools to extract data faster and feed it into downstream automation.We are also exploring role-specific co-pilots for case managers, transfer specialists, and suitability specialists.
- Product area: We continue to use traditional statistical models for price elasticity.
ROI Case for AI
Paul Tyler: How do you make the ROI case for AI investments?
Doug Elfers: First, align with the business strategy and drive metrics that support yearly goals. Don’t build AI on the side and then try to sell it – you need business alignment from the start. Regarding model accuracy, set realistic expectations – it won’t be 100% accurate. Consider the human error rate you’re replacing; if the human error rate is 5% and the AI error rate is similar, the ROI should be positive.
For calculating benefits, I use traditional frameworks from machine learning. Start with historical reporting inputs (application volume, number of calls) and inputs driven by the AI’s value. Begin with your best guess of the value, then run experiments or proof of concepts to refine that estimate. This approach helps you fail fast if needed.
Paul Tyler: Dan, you’ve been focused like a microscope on call centers. How do you think through the investment, time, and features as you build and scale what you’ve been working on?
Dan Gremmell: Scoping is crucial. We focus on problems with significant potential uplift where substantial human effort is being spent. For example, with call quality scoring, our managers would take people off phones to listen to and score calls – only reaching about 1% of our 1.5 million annual calls. By automating this process, we not only saved time but also provided quicker feedback to associates. The ROI was raising the quality bar rather than just saving time.
I agree with Doug about getting to a proof of concept quickly. Many AI solutions can be non-deterministic or difficult to control, so the faster you can prototype and see how it reacts, the better. I always push my team to develop an MVP scope to validate the concept first rather than trying for perfection.
Paul Tyler: How much could your approach change the cost of a call center per carrier?
Dan Gremmell: The change could be drastic. We’ve tested voice automation to avoid calls entirely while still allowing self-service. There are two ways to think about it: either avoiding human involvement completely (saving the entire cost) or improving the efficiency of humans taking calls. If you can reduce a call from 7-8 minutes to 4-5 minutes, you immediately create value by using less capacity.
Manu Mazumdar: Looking back 20 years when I ran part of Mass Mutual, a tech company pitched outsourcing our call center. They broke down the costs: a human answering a call cost about $10, routing through an IVR system brought it down to $3-4, and web self-service cost about $1. They offered to put humans on calls in Malaysia and India for 0.6 cents per call.
Fast forward 20 years, and countries like Malaysia and Indonesia that transformed their economies with call centers are now seeing those jobs decimated by AI agents. The cost equation comes down to the delta between a machine versus a human handling calls.
Just this morning, Workday announced they’re deploying AI agents for HR work. I joked with them that they’re taking the “human” out of human resources and replacing it with “air.” While the agentic workforce is becoming reality, we still need empathy. A recent 60 Minutes interview featured a Nobel laureate in physics who also has PhDs in neuroscience and AI. When asked how long it would take for AI models to develop empathy, he said about 36 months. It’s coming.
Buy vs. Build Decisions
Paul Tyler: Imad, you need to double your assets under management without a massive budget. How do you determine your tech stack? What do you build internally versus buy?
Imad Eid: Simplification is super important in technology – fewer systems are easier to maintain. As an insurance investment company rather than a tech company, we prefer to buy solutions when possible. Many AI models today involve hybrid solutions where you rely on someone else’s LLM, integrate through APIs, and get the outcomes you need.
We consider building only in cases where we get a true competitive business advantage, like in investment areas where we have our “secret sauce.” Considerations include cost, scalability, speed to market, and talent availability. We’ve partnered with external resources to help our internal team develop capabilities.
Paul Tyler: If you’re prioritizing, what comes first – partners, strategy, or tech?
Imad Eid: Strategy comes first, as Doug summarized. For sales support, my roadmap follows exactly what Doug described. From there, we look at the right technology to support different use cases, then determine if we have the right partners and internal talent.
Regulatory Concerns
Paul Tyler: In our highly regulated environment, how does your company avoid bias and address regulatory concerns?
Imad Eid: We focus on use cases where we have control. We ensure we’re using diverse, non-biased data when training models, avoiding features like age and gender. Our governance body ensures we follow guidelines. We stay close to regulators, educating them while listening to their concerns. We leverage frameworks that provide controls for inspection and verification.
While agentic AI is promising, we’re not there yet. Most of our use cases still rely on human-AI interaction, with humans verifying outputs, which helps address compliance concerns.
Driving Behavioral Change
Paul Tyler: I think when we ask how AI changes our industry, 10% of the answer is technology and 90% is changing behavior. What are your best practices for helping people try these tools and change their behavior?
Doug Elfers: It’s all about the end user. One problem is that use cases often get prioritized at the highest level, but specific functional requirements aren’t always gathered from end users. This means when you go live, users might say they would have prioritized different features. Engage with end users from the beginning to understand their needs. AI and data is a team sport requiring collaborative development – put on a business hat, read their standard operating procedures, and understand how they do their job.
Dan Gremmell: Education is key. Most people see AI or ChatGPT and aren’t sure how to approach it. Opening up people’s creativity helps them understand how to use AI in their work. Give them a safe space to experiment and access to sanctioned software so they don’t inadvertently share something they shouldn’t. Provide the right governance alongside training and education.
Also, measure the outcomes you’re trying to achieve with AI adoption. Is it productivity improvements? Monthly active users? Set quantitative benchmarks and be honest about adoption progress so you can refine your approach.
Manu Mazumdar: At Conning, we conduct annual research with the top 100 CEOs, CIOs, and CTOs in the insurance industry. It’s now a foregone conclusion that AI is here. Twelve months ago, when we asked how much generative AI companies were using in their work processes, it barely registered. This year, 55% of respondents said they are fully implemented in generative AI.
The question I get asked most often is, “Is AI going to take my job?” My answer: AI won’t take your job, but people who know how to use AI will take your job. We all need to get comfortable using AI.
On bias and implementation – think about inclusion. If you view AI as an inclusive tool in your repertoire, you’ll be better served. It’s here to stay, so figure out how it affects your entire value chain and work processes.
Imad Eid: Working with people closest to the work is extremely important. Recently, we developed something I was excited about, but during the pilot, users immediately gave feedback: “This is so robotic, I can’t use it.” It was a five-minute fix, but crucial feedback. Working with champions and focusing on change management is huge – if people don’t adopt the technology, it just sits on the shelf.
Manu Mazumdar: The C-suite has to champion any kind of AI or cultural change. This is a cultural shift in the world. All our lives, we’ve been told to go to school, college, and graduate school to earn a rewarding job. Now graduates worry their jobs are being done by AI tools. We have a responsibility to help frame AI as a beneficial tool across the entire spectrum.
Paul Tyler: Thank you all for coming. Please reach out to everyone here for more information.